In our latest release, version 0.11.3.25, we have added support for Sequence-to-Sequence[1] (Seq2Seq) models with Attention[2][3], and do so with the newly released CUDA 11.3/cuDNN 8.2 from NVIDIA. Seq2Seq models solve many difficult problems such as language translation, chat-bots, search and time-series prediction.
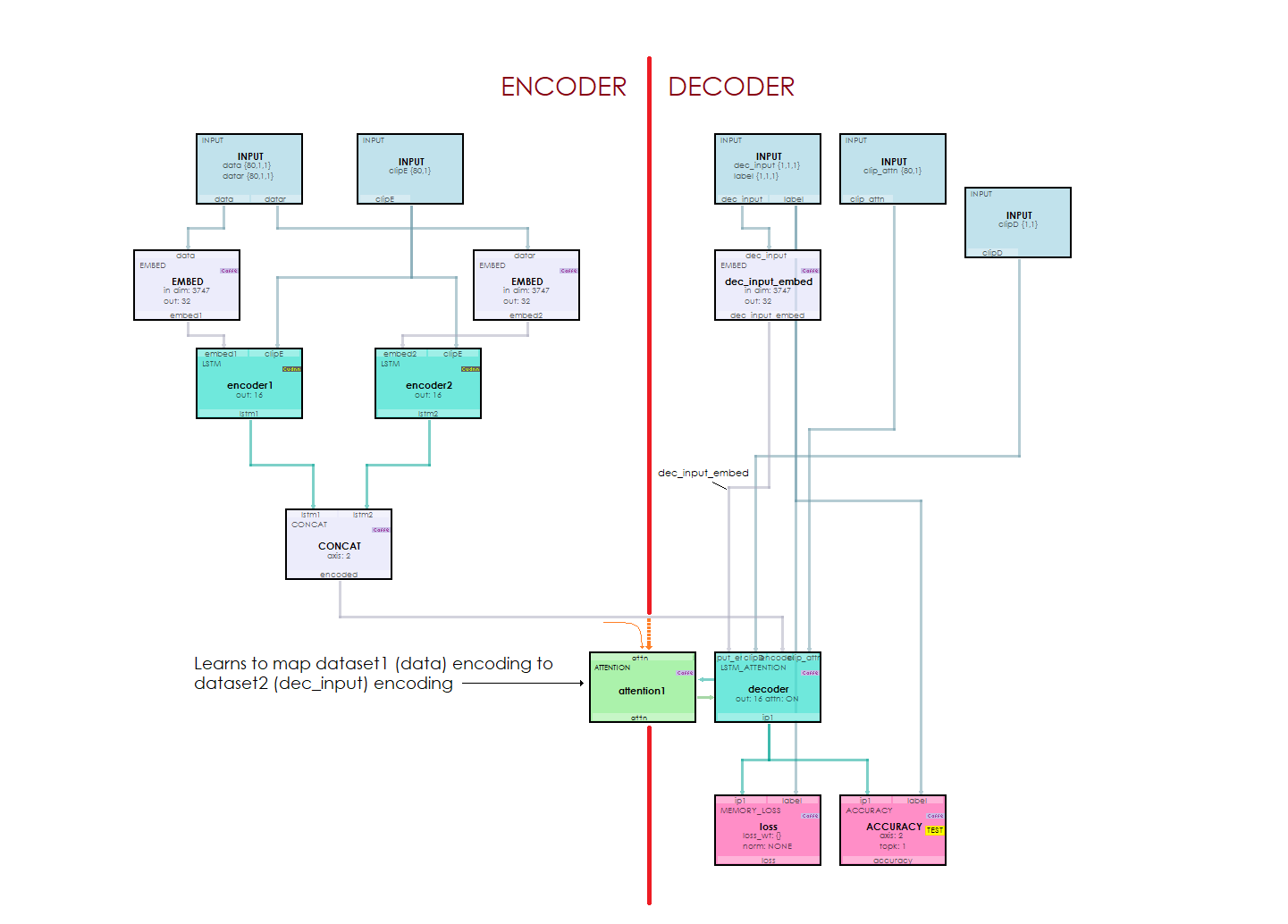
The Seq2Seq model is made up of an Encoder (left side) that is linked to the Decoder (right side) by the Attention layer which essentially learns to map the encoder input to the decoder output. During the model processing, an embedding is learned for the encoder and decoder inputs. An encoder embedding is produced for both the encoder input and its reverse representation. These two embeddings are then fed into two LSTM layers that learn the encodings for each which are then concatenated together to produce the encoding inputs that eventually are fed to the Attention layer within the LSTMAttention layer. An embedding is also learned for the decoder inputs which are then fed to the LSTMAttention layer as well.
Within the LSTMAttention layer, the encoder encodings and last state from the LSTM Attention layer are used to produce the context for the encoding inputs. This context is then added to the LSTM cell state to produce the decoded LSTM outputs which are then run through an internal inner product and eventual softmax output. The softmax output is then used to determine the most likely word index produced which is then converted back to the word using the index-to-word mapping of the overall vocabulary. The resulting cell state is then fed back into the attention layer to produce the next context used when running the decoding LSTM on the next decoder input.
During training, the decoder input starts with the start of sequence token (e.g. ‘1’) and is followed by target0, then target1, and so on until all expected targets are processed in a teacher forcing manner.
Once training completes, the model is run by first feeding the input data through the model along with a decoder start of sequence (e.g. ‘1’) token and then the decoder half of the model is run by feeding the resulting output token back into the decoder input and continuing until an end-of-sequence token is produced. The string of word tokens produced are each converted back to their corresponding words and output as the result.
This powerful model essentially allows for learning how to map the probability distribution of one dataset to that of another.
With this release, we have also released three sample applications that use the LSTM models in three different applications.
SinCurve – In the first sample, we use the LSTM model to learn how to produce a Sin curve by training the model with teacher forcing on the Sin curve data where the previous data predicts the next data in the curve.
ImagetoSign – in the next sample, we use the LSTM model to learn to match hand drawn character images from the MNIST dataset, shown in sequence, to then draw different portions of the Sin curve. The second to last inner product data from the MNIST model is input into the LSTM model which then learns to produce a segment of the Sin curve based on the hand written character detected.
Seq2SeqChatBot – in this sample, a Seq2Seq encoder/decoder model with attention is used to learn the question/response patterns of a chat-bot that allow the user to have a conversation with the Chat-bot. The model learns embeddings for the input data that are then encoded with two LSTM layers and then fed into the LSTMAttention layer along with the encoded decoder input to produce the output sequence.
New Features
The following new features have been added to this release.
- Added support for CUDA 11.3 and cuDNN 8.2 with NVAPI 650.
- Tested on Windows 20H2, OS Build 19042.985, SDK 10.0.19041.0
- Added ability to TestMany after a specified time.
- Added signal vs. signal average comparison to Model Impact Map.
- Added color separation to Model Impact Map.
- Added support for visualizing custom stages from solver.prototxt.
- Added new MISH layer support.
- Added new HDF5_Data layer support.
- Added new MAE Loss layer support.
- Added new COPY layer support.
- Added new LSTMAttention layer support.
- Added new Seq2Seq model support with Attention.
Bug Fixes
- Fixed bug in RNN learning where weights are now loaded correctly.
- Fixed bugs related to accuracy calculation in RNN’s.
- Fixed bug causing UI to lockup when multiple tasks were run.
- Fixed bug in Copy Dataset creator where start and end dates are now used.
- Fixed crash occurring after out of memory errors on application exit.
- Fixed crash caused when importing weights with missing import directory.
- Fixed bug where InnerProduct transpose parameter was not parsed correctly.
- Fixed bug in concat_dim, now set to uint?, and ignored when null.
- Improved physical database query times.
Known Issues
The following are known issues in this release.
- Exporting projects to ONNX and re-importing has a known issue. To work around this issue, the weights of an ONNX model can be imported directly to a similar model.
- Loading and saving LSTMAttention models using the MyCaffe weights has known issues. Instead, the learnable_blobs of the model can be loaded and saved directly.
Happy deep learning with attention!
[1] Ilya Sutskever, Oriol Vinyals, and Quoc V. Le, Sequence to Sequence Learning with Neural Networks, 2014, arXiv:1409.3215.
[2] Ashish Vaswani, Noam Shazeer, Niki Parmar, Jakob Uszkoreit, Llion Jones, Aidan N. Gomez, Lukasz Kaiser, and Illia Polosukhin, Attention Is All You Need, 2017, arXiv:1706:03762.
[3] Jay Alammar, The Illustrated Transformer, 2017-2020, Jay Alammar Blog.