In our latest release, version 0.11.2.9, we have added a new powerful debugging technique that visually shows the areas within an image that impact the firing of each label, and do so with the newly released CUDA 11.2/cuDNN 8.1 from NVIDIA.
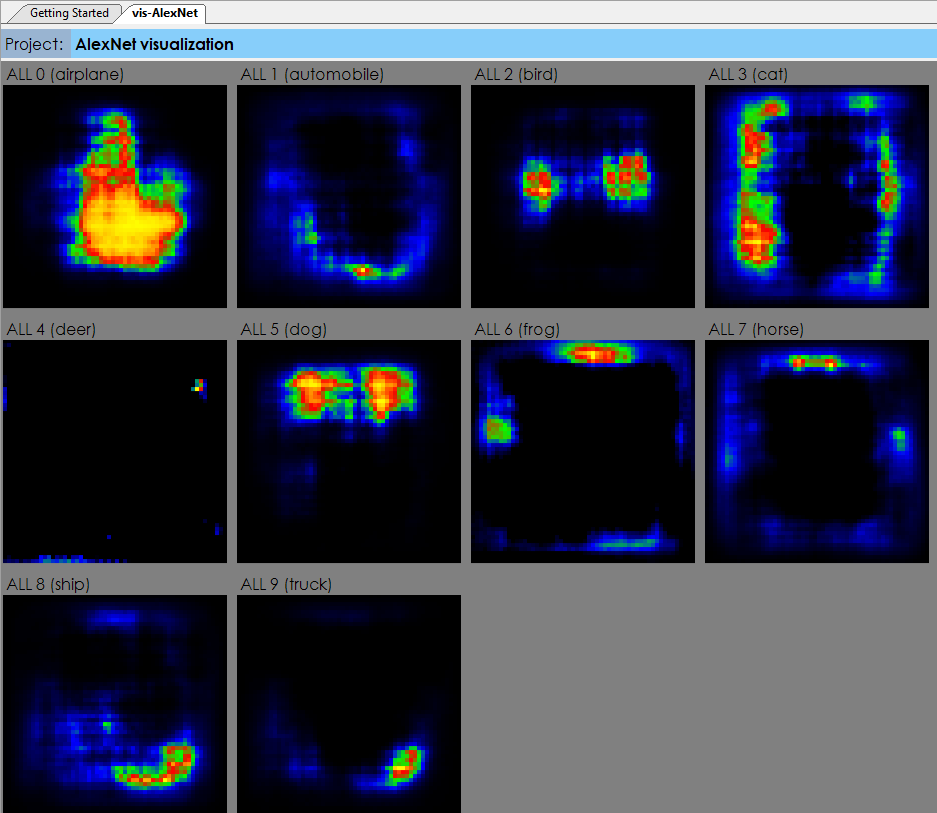
The example above shows the areas within the CIFAR-10 [1] dataset images that actually have the most impact on firing each detected label.
Unlike the label impact visualization, originally inspired by [2], the model impact visualization shows the impact of each area of the image space on all labels, whereas the label impact shows the impact on a single label.
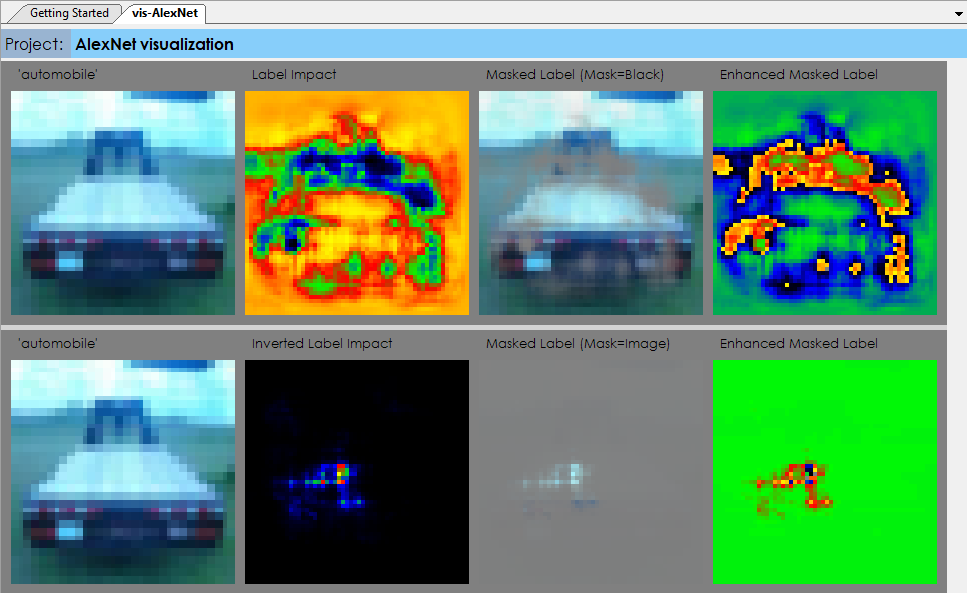
To learn more about these debugging techniques, see the new ‘Debugging AI Solutions‘ tutorial.
New Features
The following new features have been added to this release.
- Added support for CUDA 11.2 / cuDNN 8.1.
- Added new Model Impact Visualization.
- Added new Model Reverse Visualization.
- Added new Transpose Layer.
- Added new Gather Layer.
- Added new Constant Layer.
- Added ONNX InceptionV2 to public models.
- Added support for compute/sm 3.5 through 8.0.
- Added ability to reset weights in any layer.
- Added ability to freeze/unfreeze learning in any layer.
- Optimized project status updates.
- Optimized image loading.
Bug Fixes
The following bugs have been fixed in this release.
- Fixed bug related to scheduling solvers with file data.
- Fixed bug related to scheduling Char-RNN projects.
- Fixed bug related to reinforcement learning rewards display.
- Fixed bug in model editor where nodes exceeded 32k pixel limit.
- Fixed bug related to renaming data input causing failures.
For other great examples, including, Neural Style Transfer, beating ATARI Pong and creating new Shakespeare sonnets, check out our Examples page.
[1] Alex Krizhevsky, The CIFAR-10 dataset.
[2] Matthew D. Zeiler and Rob Fergus, Visualizing and Understanding Convolutional Networks, 2013, arXiv:1311.2901.